Machine Learning for Indoor Localization and Navigation, 1st ed. 2023
Coordonnateurs : Tiku Saideep, Pasricha Sudeep
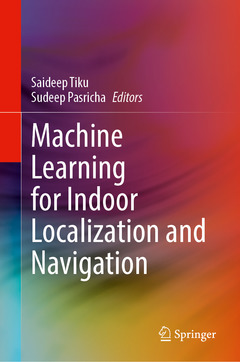
- Provides comprehensive coverage of the application of machine learning to the domain of indoor localization;
- Presents techniques to adapt and optimize machine learning models for fast, energy-efficient indoor localization;
- Covers design and deployment of indoor localization frameworks on mobile, IoT, and embedded devices in real conditions.
Introduction to Indoor Localization and its Challenges.- Advanced Pattern-Matching Techniques for Indoor Localization.- Machine Learning Approaches for Resilience to Device Heterogeneity.- Enabling Temporal Variation Resilience for ML based Indoor Localization.- Deploying Indoor Localization Frameworks for Resource Constrained Devices.- Securing Indoor Localization Frameworks.
Sudeep Pasricha is a Walter Scott Jr. College of Engineering Professor in the Department of Electrical and Computer Engineering, the Department of Computer Science, and the Department of Systems Engineering at Colorado State University. He is Director of the Embedded, High Performance, and Intelligent Computing
Provides comprehensive coverage of the application of machine learning
Presents techniques to adapt and optimize machine learning models for fast, energy-efficient indoor localization
Covers design and deployment of indoor localization frameworks
Date de parution : 06-2023
Ouvrage de 567 p.
15.5x23.5 cm
Disponible chez l'éditeur (délai d'approvisionnement : 15 jours).
Prix indicatif 116,04 €
Ajouter au panier